Technical Report
Human-Machine Team (HMT) Concepts for Resilient Autonomous Systems: Experimental Design Approach to Human-in-the-loop Simulation
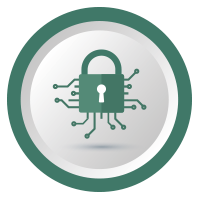
Report Number: SERC-2020-TR-016
Publication Date: 2020-10-19
Project:
Human-Machine Team (HMT) Concepts for Resilient Autonomous Systems
Principal Investigators:
Dr. Inki Kim
Co-Principal Investigators:
Dr. Stephen Adams
Dr. Peter Beling
Dr. Barry Horowitz
Background and Challenges: Through cyberattacks, adversaries can potentially create a wide range of disruptive situations that seriously degrade system performance, compromise missions, and even hamper a pilot’s situational awareness via deception techniques (such as physical decoys or corrupted information). A difficulty with creating cyberattack-resilient solutions that can support systems in a certain mission context is that such adverse situations tend to be extremely diverse and sparse, and may further evolve within the context. They thus constitute an “ill-defined” problem space in which a problem and solution pairs are not clearly established. Such a vague linkage between a problem and its solution sets also complicates military cybersecurity training, which has conventionally pursued proficiency in well-defined procedures and rule-based skills.
Rationale: The rationale for this project is that 1) cyberattack-resilient solutions frequently require adjustments to both the technical and operational (re)configurations of an attacked system, thereby necessitating the presence of operators who may possess situational knowledge that may not be available from the technical components of a system (e.g., the current context of a battlefield situation or the impact of an altered configuration on other activities); and 2) human-machine teamwork is crucial for effective responses to atypical cyberattacks having limited prior information, or for customized responses in pursuit of highly context-driven goals for mission success, where operators should learn to manage the “right” level of confidence on the advanced support of cyberattack detection and system reconfiguration.
Goal and Objectives: The current project pursues robust human-machine teamwork in providing cyberattack-resilient solutions to support continued mission operation and success, despite deteriorating circumstances due to cyberattacks and even subsequent failures of machines or humans. Contrary to the traditional, functionally oriented paradigm of automation (i.e., the machine takes over from the human, or vice versa), a breakthrough is to keep the human-machine team (HMT) as an elementary unit to handle cyberattacks while one party can learn to cover for the vulnerability of the other. Toward that vision, this project aims to examine post-cyberattack HMT performance in the mission context of unmanned aerial vehicle (UAV) surveillance on a remote mission location, through human-in-the-loop simulation experiment. Specifically, the experiment has the following objectives:
- Objective 1: Devise and validate an experimentally driven approach to examine human-machine team (HMT)’s resilient performance under cyberattack and suboptimal system operations in various mission contexts.
- Objective 2: Investigate how UAV pilots respond to the need for the technical and operational reconfigurations of disrupted system behaviors, by using a general framework of planning (i.e., setting goals, implementing solutions, and evaluating how the solutions satisfy the goals) in the context of the mission being sustained.
- Objective 3: Propose new approaches to improve resilient HMT performance, including the development of intelligent Sentinel that is aware of risk attitudes and risk behaviors of the pilot.
Overview of Experiment: Our experiment intended to examine resilient human-machine cooperation under suboptimal systems operation due to malicious cyberattacks on military assets. It focused on cyberattacks that corrupt the performance of an unmanned aerial systems (UAS). In particular, the experiment tested the pilot’s selection of recovery solutions as well as confirmatory actions under simulated cyberattacks, with the set of solutions representing the tradeoffs between the quality and time cost to the ongoing mission. The pilots were recruited at the AFIT and oriented on the concept of resilient HMT and cyberattacks (i.e., how cyberattack-resilient solutions could impact system recovery), about basic UAS components (including Sentinel), and how to control interfaces. And when a simulation-in-the-loop test began, the participant was briefed about the mission. At the end of each mission scenario, the participant logged in an online survey to record their own reasoning and response options selected for the specific mission scenario being tested, including how tradeoff decisions were made and the confidence in making those decisions.
Intellectual Merit: Today, emerging cybersecurity systems for countering cyberattacks are intended either to replace, or be replaced by, humans when disruptive threats emerge. That approach fails to take advantage of human-and-machine synergies that could have enabled more resilient systems. The key hypotheses examined in our project were: 1) that human-in-the-loop mission simulation is effective to test resilient HMT performance under disruptive system events, and 2) in those mission scenarios tested, HMT outperforms both operator without system support (i.e., Sentinel) and system reconfigurations without operator, in terms of the quality of the resilient solutions selected for the scenarios. For future research, the project will show that the superiority of the HMT performance will be guaranteed if the system is aware of operator’s risk attitude and risk behaviors (through AI driven approaches), for which the human-in-the-loop simulation will allow the system to learn operator characteristics under various mission context.
Broader Impacts: The proposed human-in-the-loop approach to measure resilient HMT performance will allow military personnel to experience diverse symptoms/ signs of cyberattacks and the pros and cons of reconfiguration options without imposing risks to the mission. In addition, maintaining an appropriate level of suspicion about the system throughout the mission operation even when no alert has been issued (also, not blindly following system-generated recommendations when they interfere with the mission context) will be an important benefit of simulation learning. That learning paradigm can be extended to other performance-critical workforce training domains, in which the use of AI has been controversial because of either too much or too little confidence on human roles. Still, there may be an unavoidable gap if the post-cyberattack responses would be carried out in real-world ground control missions. Controlling for the levels of immersion, interactivity, and complexity will be crucial to minimizing that gap, and we must succeed in doing so or risk invalidating the research findings.