Technical Report
Developing the Systems Engineering Experience Accelerator (SEEA) Prototype and Roadmap – Year 3
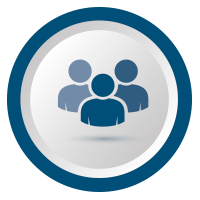
Report Number: SERC-2013-TR-016-3
Publication Date: 2013-12-31
Project:
System Engineering Experience Accelerator (SEEA)
Principal Investigators:
Dr. Jon Wade
Co-Principal Investigators:
Dr. William Watson
This document is a summary of the work that was completed in the second increment year of the SERC Research Topic DO1/TTO2/0016 “Developing Systems Engineering Experience Accelerator (SEEA) Prototype and Roadmap” supported by the Defense Acquisition University (DAU). The purpose of the research project is to test the feasibility of a simulated approach for accelerating systems engineering competency development in the learner. The SEEA research project hypothesis is:
By using technology we can create a simulation that will put the learner in an experiential, emotional state and effectively compress time and greatly accelerate the learning of a systems engineer faster than would occur naturally on the job.
- Project Management - Inability to support known and evolving customer/user feedback with current staff, budget and timeframe.
- Configuration Management – Inability to successfully manage the large number of files, configuration variables, present in the Experience Accelerator. Report No. SERC-2013-TR-16-3 December 31, 2013 iii
- Technology Development - Inability to tradeoff long-term architecture and technology objectives (leading to successful open source support) vs. short-term prototype goals.
- Content Development - Inability to produce a prototype that provides a compelling experience, supports the desired learning and is seen to be authentic.
- Evaluation - Inconclusive results due to threats to validity of Experimental design (inability to generalize results), limited availability of suitable subjects and insufficient literature to support development of evaluation instruments.
A set of lessons learned was compiled and categorized as noted below:
- Competencies, Learning and Content
- Complexity/Effort vs. Authenticity/Learning
- Technology 4. R&D Processes
A DAU student pilot review was held on October 29-30, 2013 with the following sponsor representatives: James Anthony, Tony Costanza, Darren Dusza, Steven Jones, Scott Lucero, Dave Pearson, and John Snoderly. Despite a number of technical issues relating to networking capabilities and application stability and shortage of class time, the potential of the Experience Accelerator was validated through feedback from the students.
A number of the targeted lessons were clearly represented in the team presentations, an indication of the effectiveness of the EA in promoting its targeted learning outcomes. For example, for problem solving and recovery, the importance of small schedule delays and the use of additional staff to remediate the schedule problem was touched on by several teams. Teams 1, 3, and 5 mentioned the need to hire staff early in their lessons learned, while Team 2 called for more dramatic staff shifts. Team 4 highlights the results of slipping the schedule too much, lamenting that they were fired for slipping CDR.
Another example can be seen with “Cutting corners to make short term goals while ignoring long term outcomes” which stresses the need to make decisions early. This was touched on repeatedly by the teams in their lessons learned. Team 1 mentions hiring more staff early, Team 3 calls for shifting staff earlier, Team 4 notes how their early emphasis on software worked, and Team 5 reflects on the need to ramp up staff more quickly.
These examples demonstrate that for these two targeted learning outcomes in particular, nearly all of the teams learned the outcomes as they very clearly highlighted them in their presentations as the lessons they had learned. Other learning objectives were also highlighted by the different teams. These lessons were learned despite the fact that the learners only fully completed the first two phases of the experience before speeding through the remaining phases in order to see their results. Furthermore, the EA was designed to be played multiple times by learners, so these results are indicative of impressive learning gains given the limited implementation of the experience.
Students also were asked to provide their perceptions of the EA – what it did well and what could be improved. The class discussed these and the comments were captured. Comments highlighted a number of key features of the EA as positives. These included the case-based format of the EA, noting its representation of real-life issues and modeling of real work interactions. Students also noted the importance of immediate feedback on the decisions and the interactive nature of the simulation – accelerating learning by simulating a project lifecycle in a short amount of time. The challenging aspect of the simulation was also highlighted as it was noted that being fired kept the learning challenging a more interesting, a possible reference to the EA’s targeted “scar tissue” – emotional connection in order to promote learner transfer of learned objectives. One key positive from the feedback was that the user interface received a great deal of praise – an important change from the SME feedback.
Recommendations provided additional insights on how we can further improve the EA. For example a greater focus on performance and technical aspects as opposed to cost and schedule was highlighted. A few small bugs were also identified which will be corrected, and information which will be included in future iterations of the instructors’ manual will help to further improve the efficacy of the EA.
Ultimately, the formal evaluation of the EA was hindered by the inability to compare novice learner actions and thinking to that of expert SMEs. However, while this comparison could not be made in this implementation, it certainly could be done in a future implementation. Furthermore, clear evidence of learning was gathered from the data that was gathered and learner perspectives on the efficacy of the EA were largely positive while still providing some helpful suggestions for improvement. The formal evaluation can therefore be seen as a success and indicative of the EA’s efficacy in meeting its targeted learning objectives.
Follow-on work has been defined for Increment 3 that is focused on the following:
• EA System Capabilities
o Completion and stabilization of multi-learner mode
o Provide means of informing learner of impact of recommendations
o Ensure that dialog is synchronized with recommendations
o Improve learner interface with status charts to eliminate need to page through entire set
• Tools
o Create set of tools that allow the DAU to customize and create new Experiences
• Deployment Deliverables
o Define explicit EA deliverables to support DAU deployment
• Hosting Requirements
o Specify technical details of hosting requirements